Meniscus received news this week they are through to the short-listed stage of the CivTech ® Pilot 2016.
The CivTech® Pilot is seeking new technologies to drive bold innovation in the public sector. It brings together private sector innovation, public sector organisations and citizens to develop more efficient and effective products and services, which will translate to new, better, faster and easier experiences for everyone.
Backed by the Scottish Government, it is going to provide an unprecedented route for entrepreneurs, start-ups, SMEs and other businesses to develop the benefits of digital transformation in the public sector, creating an opportunity for businesses to win public sector contracts.
The first of the six-part challenge process saw all 85 applicants completing their application to determine the overview of their idea, their level of innovation, and commercial potential. The second stage in the process, the Exploratory stage, included assessments on the participant’s business acumen, team and exploratory skills.
Meniscus Systems Limited has successfully reached the third stage of the selection process and is hoping to provide a potential, and successful, solution for challenges taking them into the Accelerator stage where they will work to further develop their potential solution, engaging closely with the Challenge Sponsor and the CivTech ® pilot team, further establishing the viability of their potential solution, and address any issues that have been brought up.
The six Challenges are simply a problem which a Challenge Sponsor (public sector organisation) would like solved. CivTech® pilot is focusing on the following areas.
Challenge 1: How can we help improve air quality in urban areas?
Challenge 2: How can we make our flood forecasting information better used by a wider audience?
Challenge 3: How can we get health and social care data and analysis to the widest possible audience?
Challenge 4: How can we make our data publications more accessible and appealing?
Challenge 5: How can we promote the tourist destinations along the A9?
Challenge 6: How can we use technology to design smart roads?
Mike Everest, Managing Director and owner of Meniscus Systems Limited, commented, ‘We are delighted to have been shortlisted in two very diverse and exciting markets, challenge 1 and 6, namely Air Quality work and a SMART Road project for the A9 dualling. This is a long-term development of the A9 as a dual carriageway.’
Mike further said, ‘We have a pitch to present next week to see if we make it to the next stage of the challenge, which will be the Accelerator stage where an initial implementation of the project takes effect to start to meet the needs of the challenge.’
Civtech® will announce the Accelerator finalists on 5 September 2017.
Download the Hyperlocal Rainfall App on Android and iPhone
Meniscus Systems have officially released the Hyperlocal Rainfall App onto iTunes. Users can now download the app on: Android – Google Play iOS – iTunes The simple-to-use ‘Hyperlocal Rainfall’ App uses GPS location for point-to-point journeys, to provide highly-localised rainfall predictions for imminent time-windows, allowing users to plan their journeys. Using the App allows them to undertake […]
Automated invoice generation and management reporting for CHP units
Meniscus was recently approached by a power company to create an automated invoice generation and management reporting service for their CHP units. The invoices will be based upon raw data sent to the Meniscus servers and include using a range of key variables and unit rates that will be entered into a simple bespoke web page.
MAP came to the fore as it is specifically designed to be both scalable and very flexible. A comprehensive RESTful API is available that lets users configure every element of the underlying database and to add or delete and update any element of the platform. This API is used to build a mobile friendly website that provides configuration capability, analytics, widget graphs and a data import facility.
Because MAP is deployed on a variety of cloud servers it means any new server instances can be readily available online as users require.
The main benefits when using the Meniscus Analytics Platform (MAP) are:
Ø It enables integration and automation of more complex analytics directly into your services.
Ø It provides a means to rapidly develop and deploy new solutions and services.
Ø Provides flexibility to change the analytics being delivered as a solution matures and evolves.
Ø Provides a means to reliably scale, from a pilot scale up to a full-scale project, quickly and easily.
Ø It is a cost effective way for delivering complex analytics.
Setting up the database
Meniscus will be setting up the database to deliver the invoice information using the actual data uploaded from each CHP unit that uploads data to the Meniscus servers.
An initial ‘template’ site will be created using the most complex tariff structure envisaged. The invoice data from this one site can then be used to test the calculation accuracy using a range of input variables and a range of imported raw data. Once this ‘template’ site is verified then it will be used as the basis for all other CHP sites.
The validation process will be replicated in case changes are required to this template site at a later date.
Importing data
Meniscus will create a bespoke importer to convert the CHP data into the required format for import into MAP.
Bespoke dashboard
Meniscus will set up a simple yet secure ‘one-page’ dashboard to enable staff to enter and update key variables and unit rates as and when required.
Management reporting
Meniscus will set up two management reports. These reports will be run on a scheduled basis (e.g. daily, weekly or monthly).
Formatted output
Meniscus will collate and format the output data into an agreed format which will be forwarded at the end of each month and will form the basis of the invoice data.
Hosting Services
Specific Hosting Services
Ø Processing data received to create the required output invoice format. Raw data files will be uploaded from the CHP units at daily intervals and MAP will automatically process all the available data. This effectively means that it will be possible to view invoice data for the current month at any point in time.
Ø Delivering scheduled management reports.
General Services
Ø Provide server facilities that include the appropriate data backup and failover capability.
Ø Provide telephone and e-mail support.
Ø To carry out reasonable minor updates and changes to the database and associated calculations if requested by the customer.
Identifying the risk of a flood at specific locations in your area?
Meniscus provides software that gives a “heads up” about the risks of flooding at a specific flood risk location. Our service brings together a number of sets of data into one easy to use dashboard and calculates a range of metrics that identify if there is a potential flood risk.
Do you have locations where an early warning of a potential flood risk would be beneficial?
Would a visual overview of likely rain events and any potential flood risk be helpful to you? If so, this is how it works:
Meniscus software uses and combines a range of data source sets and collates these into one simple dashboard to monitor and predict likely rainfall levels to provide early warning signs of potential risks. The information displayed in the dashboard includes:- Current rainfall (updated every 5 minutes)
- Forecast rainfall for the next 36 hours
- Antecedent Precipitation Index (API) to calculate ground saturation – uses the last 30 days of rainfall (updated every 5 minutes)
- Maximum forecast rainfall today and tomorrow
- Maximum Rainfall Return Period (1 in 5 year rain event) for today and tomorrow
- River level – if appropriate and if it is monitored by the Environment Agency (updated every 5 minutes)
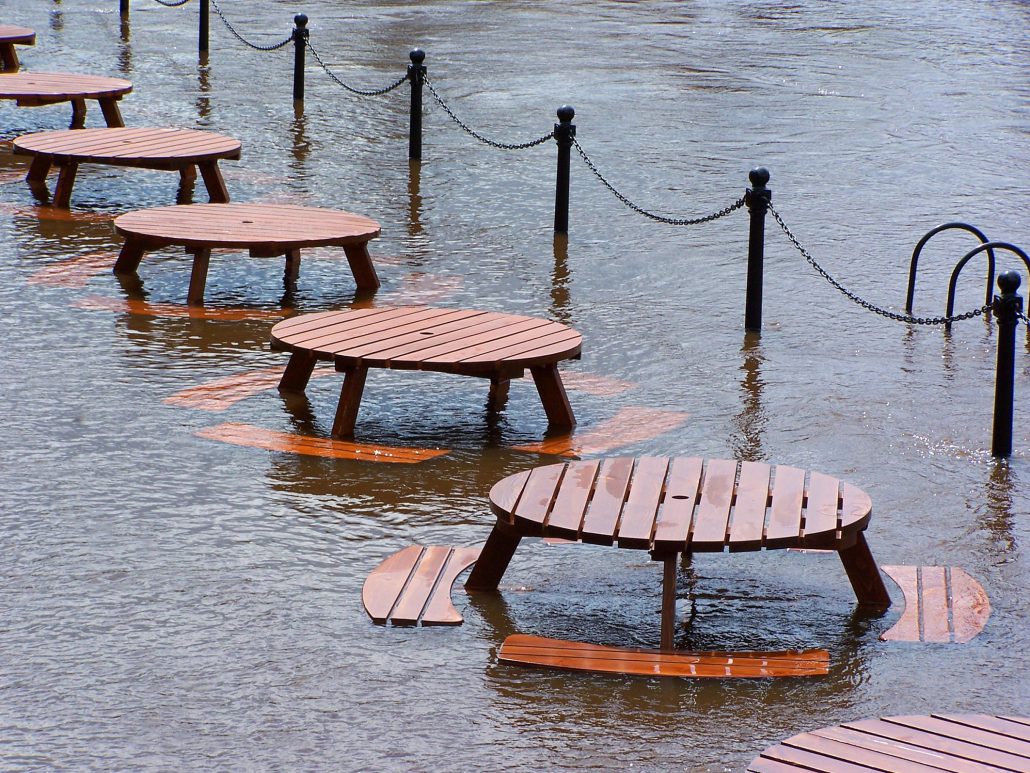
The Environment Agency gauging station river level data is an open dataset and we use the Environment Agency flood and river level data from the real-time data API (Beta)
River Ouse burst its banks in community picnic area
Flood Forecasting part 2
A new project has been undertaken by several UK Councils using MAP to deliver specific localised flood monitoring and is currently being tested by Cambridge City Council.
Flood forecasting
This service combines actual radar rainfall and forecast rainfall data and uses it to calculate the maximum rainfall return period for a specific location for the next 36 hours as well as the Antecedent Precipitation Index (API) as an indicator of moisture levels in the soil (this calculation uses soil type data).
The solution also displays river level gauging data from the Environment Agency and the historic and forecast information is displayed in a mobile-friendly dashboard. The next stage in this project is to implement a learning based model to correlate increases in these rainfall metrics with the increases in the river gauging height.
All the above working examples demonstrate some of the capabilities of MAP in terms of integrating a broad variety of datasets into models built inside MAP as well as the capability to use MAP to deliver aggregated datasets into third party models run externally to MAP.
MAP makes extensive use of RESTful Web Services making it possible for approved third parties to easily load new datasets, create new Entities and extract aggregated/calculated datasets from MAP. An Entity is any unique identifiable “Thing” which in this context might be a community, a property, a catchment etc. Each Entity contains internal ‘hooks’ to the calculations/models required to turn any raw dataset into the required calculated metrics. MAP provides the framework to allow these Entities to be readily extended making it easy to change the underlying models/calculations and adding in new database properties and metadata.
It is also envisaged that MAP will use additional datasets to enhance the capabilities of existing models and potentially use these to improve the inputs into current models. These additional datasets might include:
It is envisaged that a final solution may comprise an enhanced mobile app and associated mobile friendly dashboard delivering location specific flood information derived from this broad range of datasets. Importantly this flood information will use machine learning to improve flood predictions based on the relationship in the actual data and will not rely upon complex deterministic models – unless they are already available.
How Innovative is MAP for Predicting Flooding?
Predicting Flooding
Flood Prediction
Existing solutions for predicting flooding are heavily reliant on the use of complex models that are very expensive and time-consuming to build. In addition, these models can be too complex to readily run in real time conditions.
The Meniscus approach looks to replace this by using existing datasets and models wherever possible to build up simplified models that can be run in real time.
These simplified models can then be improved upon using a combination of machine learning and by using additional datasets (like satellite imagery) to improve the assumptions and variable on which the models are built. Consequently, this approach is ideal for delivering real-time flooding information for a large number of relatively small catchments (it is assumed that the existing more complex models will cover known flooding hotspots).
The use of the algorithms already developed in MAP to track existing rainfall and predict its course over the next hour will enable the solution to rapidly respond to the development of short summer high-intensity rain events rather than relying on forecasts that are notoriously poor at forecasting such events.
MAP itself is a new and highly innovative platform (the development was initially part funded by InnovateUK in 2014) and its structure is specifically developed to allow rapid development and changes to the underlying database structure and models. It is cloud based with a comprehensive RESTful web service interface making it possible for third parties to interact with it.
Flood Forecasting part 1
MAP-Rain is an existing flood forecasting and rainfall prediction solution based on the proven Meniscus Analytics Platform (MAP) which is a high performance, generic, Big Data, cloud based real time calculation/analytics platform.
MAP-Rain is a generic and initial solution for flood forecasting and integrates additional datasets and models from the existing systems, APIs and databases already used and developed as well as a range of new datasets.
MAP-Rain delivers the following real-time flood forecasting solutions:
flood forecasting
MAP-Rain is also being used to as part of an InnovateUK funded Smart City project (Hyperlocal Rainfall) looking to increase the use of sustainable transport in cities. This solution predicts the path of actual rainfall over the course of the next hour, at five-minute increments, and relates this to specific journeys that users can create. The aim being to increase the use of cycling and walking by answering the question, “Will it rain during my journey”? This solution uses a combination of real-time radar rainfall data, local wind speed and direction data from an existing local network of weather stations and high altitude wind forecasts. The radar rainfall data is also being ground truthed to local rain gauge data to increase accuracy. As part of this Hyperlocal Rainfall project Meniscus has developed an Android mobile app for users to create journeys and to track and plan journeys around rainfall. MAP-Rain is integrating a third party personalisation engine developed to learn users’ behaviour and to personalise the app based on insights learnt by the engine. The project is initially focused around Peterborough but is also being tested using the entire radar rainfall dataset for England and Wales.
Hyperlocal Rainfall Predictions
Rainfall prediction app
Our innovative Hyperlocal Rainfall app has recently been published on the Playstore. We have had 5* reviews and between 100 and 500 installs in less than one month.
We believe the positive reviews are predominantly down to our highly accurate and hyper-localised rainfall predictions. These predictions are updated every five minutes to give 12 new rainfall predictions at five-minute intervals for the coming hour. The predictions are made using a combination of data sets including radar rainfall, wind speed and direction and rain gauges.
The ability to provide such accurate hyper-localised predictions can be somewhat attributed to ground truthing. A lot of weather predictions are based solely on radar rainfall data and although this is accurate to some extent; the radar rainfall data does not usually match the rainfall data that fell on the ground beneath the radar image. This is due to conditions such as wind speed and direction, topography etc.
The benefit of ground truthing radar data is that based on the relationship between historic radar and ground rainfall data, an equation can be derived for any location to manipulate the radar data into more closely matching the ground data. Ground-truthing significantly contributes to enabling us to deliver these highly accurate hyperlocal predictions.
Multiple Regression Analysis Lowers Fleet Costs
Do you have a fleet management system? Does this calculate how much fuel is being consumed by each vehicle and by whom? This is very important information and very often nothing, or not enough, is being done with your information.
With the constant fluctuation in fuel prices, it can be difficult for transport companies to budget their fuel costs as accurately as possible. Many factors contribute to the total fuel cost.
Multiple Regression Analytics lower fleet fuel
Lowers Fleet Costs
Meniscus has used these factors to model the cost of fuel for transport companies. Through Multiple Regression Analysis, an 11% reduction in total fuel costs could be achieved, by reducing the number of idling hours of drivers and their harsh driving scores by 20%.
Multiple Regression Analysis allows a correlation between a dependent variable and many independent variables. The analysis provides the coefficients for each variable giving an equation in the format of y = β0+ β1X1+ β2X2 +…+βnXn. From the established regression equation, it is then possible to use the independent variables to estimate the dependent variable, in this case, fuel cost. Multiple Regression Analysis also allows us to determine the contribution each individual independent variable makes to the dependent variable value. We are in the early stages of analysing transport data and believe it is a sector that would see real benefit from our MCE (Meniscus Calculation Engine).
We are not saying you shouldn’t use the system you have. All we are saying is that maybe we can do more with data and help you become even more cost efficient. In other words, get more from your system and by analysing the data produced to reduce costs, giving you more time to focus on other pertinent areas of your business.
Watch this space for new amazing on developments.
Meniscus Systems Limited Shortlisted in Selection Process CivTech
Meniscus received news this week they are through to the short-listed stage of the CivTech ® Pilot 2016.
The CivTech® Pilot is seeking new technologies to drive bold innovation in the public sector. It brings together private sector innovation, public sector organisations and citizens to develop more efficient and effective products and services, which will translate to new, better, faster and easier experiences for everyone.
Backed by the Scottish Government, it is going to provide an unprecedented route for entrepreneurs, start-ups, SMEs and other businesses to develop the benefits of digital transformation in the public sector, creating an opportunity for businesses to win public sector contracts.
The first of the six-part challenge process saw all 85 applicants completing their application to determine the overview of their idea, their level of innovation, and commercial potential. The second stage in the process, the Exploratory stage, included assessments on the participant’s business acumen, team and exploratory skills.
Meniscus Systems Limited has successfully reached the third stage of the selection process and is hoping to provide a potential, and successful, solution for challenges taking them into the Accelerator stage where they will work to further develop their potential solution, engaging closely with the Challenge Sponsor and the CivTech ® pilot team, further establishing the viability of their potential solution, and address any issues that have been brought up.
The six Challenges are simply a problem which a Challenge Sponsor (public sector organisation) would like solved. CivTech® pilot is focusing on the following areas.
Challenge 1: How can we help improve air quality in urban areas?
Challenge 2: How can we make our flood forecasting information better used by a wider audience?
Challenge 3: How can we get health and social care data and analysis to the widest possible audience?
Challenge 4: How can we make our data publications more accessible and appealing?
Challenge 5: How can we promote the tourist destinations along the A9?
Challenge 6: How can we use technology to design smart roads?
Mike Everest, Managing Director and owner of Meniscus Systems Limited, commented, ‘We are delighted to have been shortlisted in two very diverse and exciting markets, challenge 1 and 6, namely Air Quality work and a SMART Road project for the A9 dualling. This is a long-term development of the A9 as a dual carriageway.’
Mike further said, ‘We have a pitch to present next week to see if we make it to the next stage of the challenge, which will be the Accelerator stage where an initial implementation of the project takes effect to start to meet the needs of the challenge.’
Civtech® will announce the Accelerator finalists on 5 September 2017.
The Unspoken, Gaps in Data
Over the years we have come across a number of problems when it comes to receiving data. One of the common problems is where there is are gaps in data. This can cause problems for the energy management system that you may be using, and make your job even more difficult and time-consuming than it already is.
When there is no data. What do you do?
The most used and straight forward method is to carry forward values. Meaning you’re taking a previous value and bringing it forward to fill the gap. This can work when you have a single value missing and it may be sufficient depending on the scope of your needs.
Unfortunately, there is no right or wrong method of filling gaps, as it is always a compromise. The method you choose is dictated by the quality of the data you require versus the effect to attain it and the importance of the impact that the choice of the method makes to your results.
Using the MCE (Meniscus Calculation Engine) we have come up with a system that allows us to extract the data from your Energy Management System (or any other system that you may use) and pull it into MCE. This is where we set up calculations that automatically identify the data gaps.
There are various different methods filling gaps in data and there is no right or wrong method of filling gaps, as it is always a compromise. The following methods are just some of the ways you could fill the gaps in data:
Other more complicated methods involve the following:
Using MCE (Meniscus Calculation Engine), we extract the data into our systems where the calculations are set up to automatically identify the data. E-mailed Management reports are generated by MCE and the information is sent to you or directly to the Data Collector to ensure that the gaps are filled with the correct data.
Mind the Gap